AutoQuant X3 GPU and Additional Modules
Expand the capabilities of AutoQuant X3
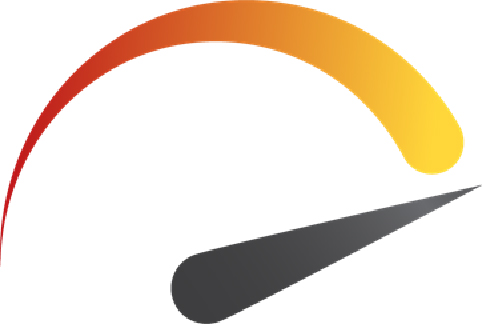
AutoQuant X3 GPU
AutoQuant X3 Deconvolution GPU Module
Optimize Your Time
Deconvolution Times vs. Image Size
Faster Processing, Same Quality
The best known and most trusted image deconvolution package is now the most affordable Graphics Processing Unit (GPU) based platform on the market. Utilizing the GPU rather than the Central Processing Unit (CPU), will allow the user to experience the same quality results in only a fraction of the time.
Upgrade your existing AutoQuant X3 package or find out how to purchase your copy of AutoQuant X3 GPU.
Easy Implementation
Faster Response, Same Easy to Use Interface
AutoQuant X3 Deconvolution GPU Module delivers even faster response times than AutoQuant X3 without sacrificing the ease of use that is inherent in the AutoQuant X3 Platform. This removes the need for extensive additional training of users and removes the downtime to obtain immediate results.
With a single click, switch between CPU based to GPU based deconvolution, increase your response time, decrease your training time, and get the full benefit of AutoQuant X3 Deconvolution GPU module.
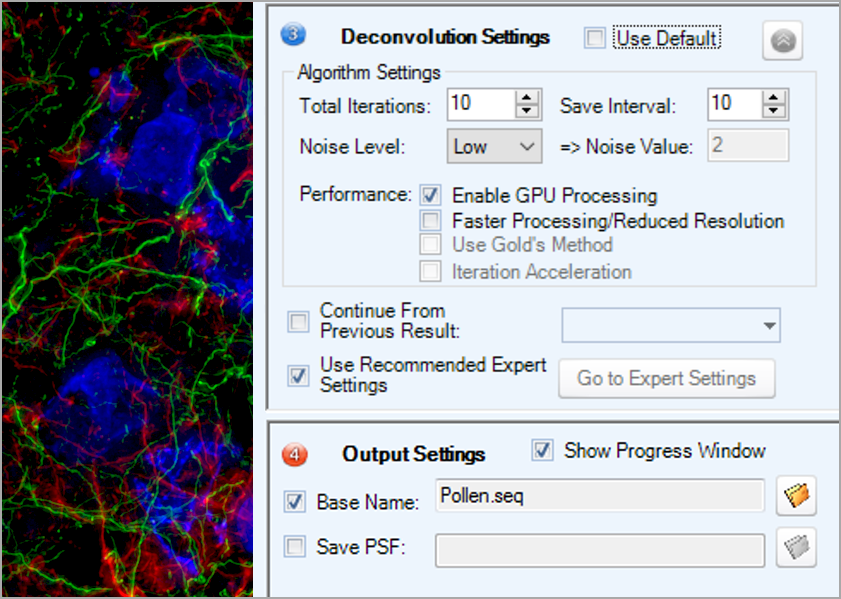
Modality | Widefield | Confocal | Confocal and Widefield |
---|---|---|---|
Brightfield | ![]() |
![]() |
|
Widefield Fluorescence | ![]() |
![]() |
|
Structured Illumination | ![]() |
![]() |
|
Light Sheet (SPIM) | ![]() |
![]() |
|
Confocal Fluorescence | ![]() |
![]() |
|
Multi-Photon | ![]() |
![]() |
|
Spinning Disk | ![]() |
![]() |
|
STED | ![]() |
![]() |
= Supported in Module
= Measured PSF
GPU Deconvolution
Understanding How it all Works
Although many in the industry refer to this as GPU based deconvolution out of necessity, certain portions of the deconvolution process still occur on the CPU, notably the initial setup. Once this is complete, AutoQuant X3 accesses the many GPU processor cores that carry out the actual iterative deconvolution. The final results are then transferred back to CPU and saved.
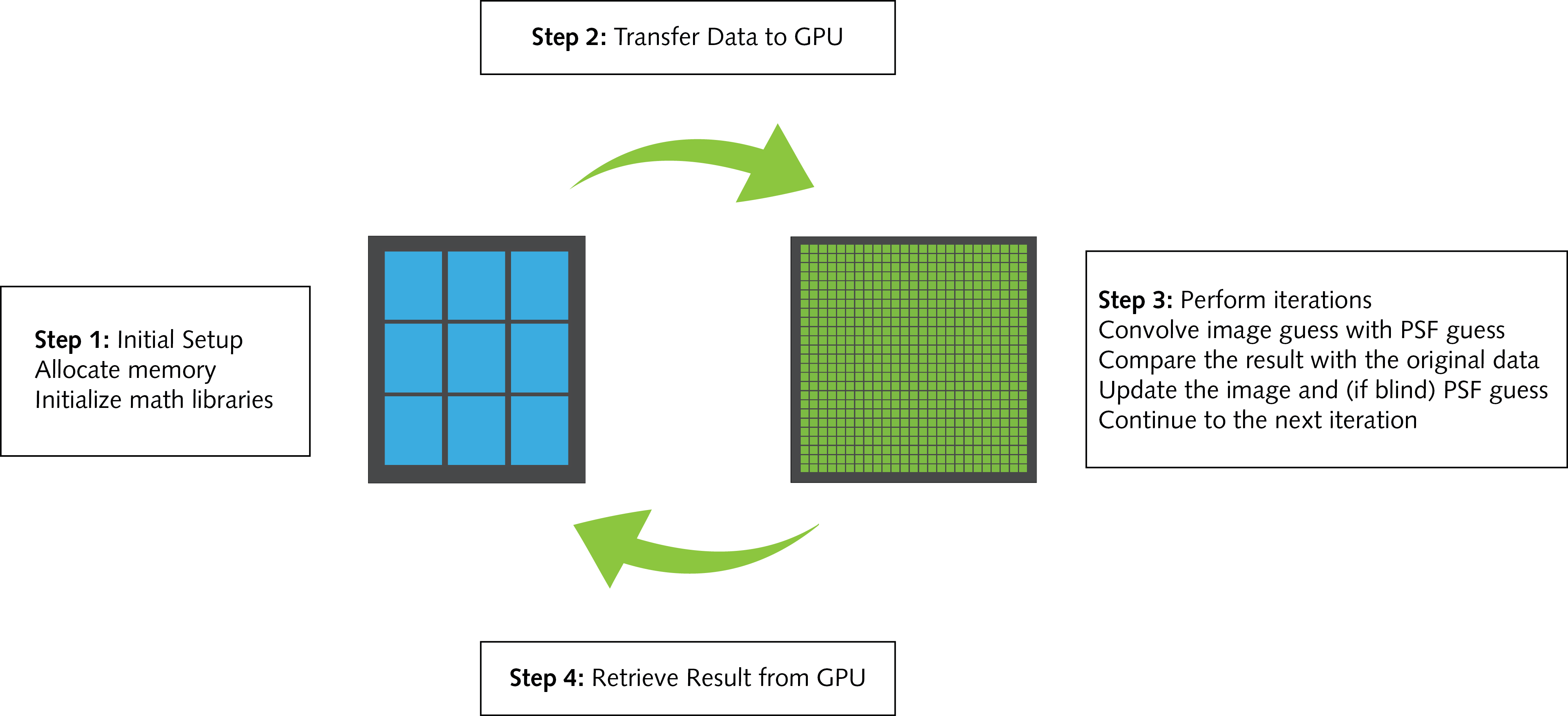
Graphics Processing Units (GPUs) have evolved from simple display adapters into computational powerhouses for applications like image processing. As rendering demands have increased, specialized processors on the GPU became more generalized, faster, and more numerous. These changes culminated in the rise of general-purpose computation being performed on devices that had nominally been designed to make special effects in games and movies look as realistic as possible.
The individual processor cores on a GPU are considerably slower than most CPU processor cores, but what they lack in power, they more than make up in numbers. The core count on a high-end workstation’s CPU(s) will be typically be around 32. In contrast, the core counts reported on even consumer-grade GPUs number into the thousands.
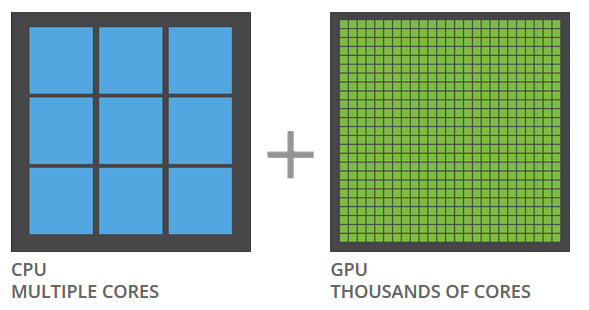
For computationally-intensive applications like image deconvolution, in which a series of mathematical calculations must be performed on each one of millions of individual voxels, being able to process thousands at once is preferable to processing a few at a time. In short, you have more bandwidth available when you utilize the GPU rather the CPU allowing for faster processing of the image.